Integrating Artificial Intelligence (AI) and Machine Learning (ML) in health care has significantly impacted the industry’s reimbursement landscape. These technologies have generated transformative changes, enhancing efficiency, accuracy, and decision-making processes within the reimbursement framework, as discussed below.
- Efficiency and Accuracy: AI and ML algorithms streamline reimbursement by automating traditionally time-consuming and error-prone tasks such as claims processing, coding, and documentation. As a result, the administrative burden on health care providers is reduced, leading to quicker reimbursement cycles and decreased likelihood of errors.
- Fraud Detection and Prevention: ML algorithms are adept at identifying patterns and anomalies within large data sets. In the realm of health care reimbursement, these technologies play a crucial role in detecting fraudulent activities. ML models can identify irregularities by analyzing historical claims data and flagging potentially fraudulent claims for further investigation. This proactive approach helps minimize fraudulent reimbursements and ensures funds are appropriately allocated.
- Clinical Documentation Improvement (CDI): AI-driven solutions help health care providers improve the quality of clinical documentation. Accurate and comprehensive documentation is crucial for reimbursement, as it directly impacts code assignment and billing. ML algorithms can analyze clinical notes, suggest improvements, and ensure that the documentation aligns with coding and billing requirements. This enhances reimbursement accuracy and reduces the likelihood of claim denials due to incomplete or inaccurate documentation.
- Risk Adjustment and Predictive Analytics: AI and ML enable advanced risk adjustment models and predictive analytics that can analyze patient data to predict future health care costs and assess the risk profile of patient populations. This proactive approach allows payers to adjust reimbursement rates based on the expected health care needs of specific patient groups, promoting fair and accurate compensation.
- Adaptive Reimbursement Models: ML facilitates the development of adaptive reimbursement models. These models can evolve over time based on real-time data and feedback, allowing for more dynamic and responsive reimbursement strategies. As health care landscapes change, adaptive models ensure that reimbursement mechanisms can quickly adjust to new trends, policies, and practices.
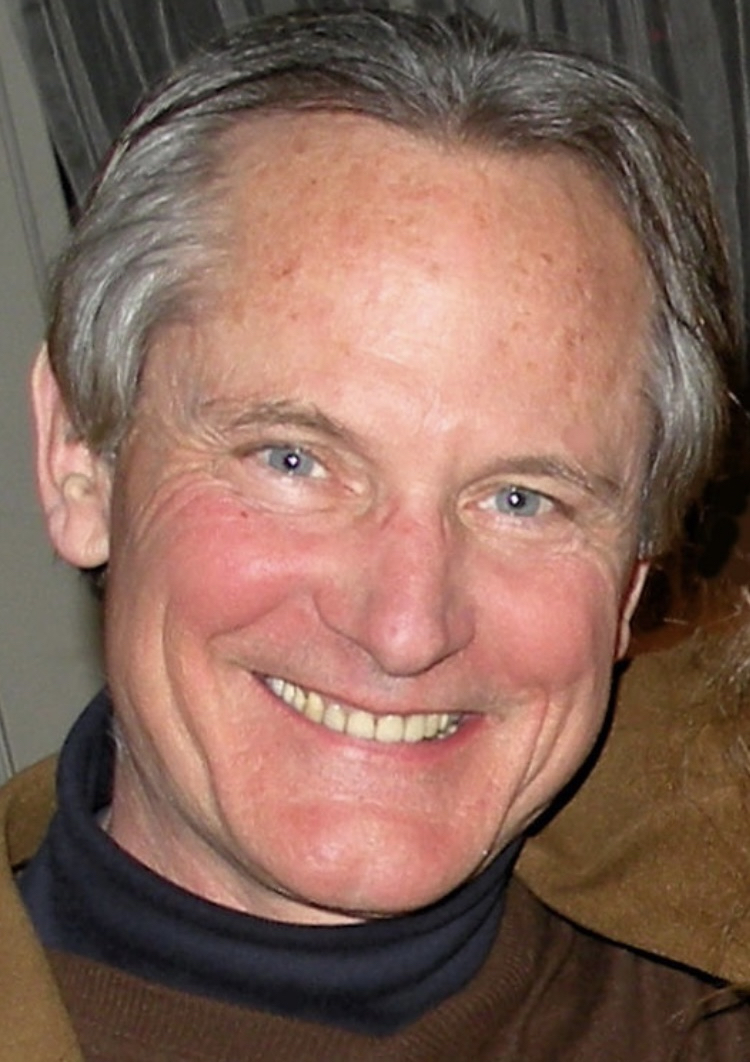
Matt Walton, former Chief Development Officer at Datacast Technologies, Inc., and past President and CEO at High Watch Recovery Center
To learn more about how AI/ML will continue to push limits with respect to reimbursement methodologies in the arena of behavioral health, including substance use disorder (SUD) treatment, I met with Alliance for Addiction Payment Reform participant Matt Walton, the former Chief Development Officer at Datacast Technologies, Inc., and past President and CEO at High Watch Recovery Center, to discuss his experience and future vision for how AI and ML will continue to impact behavioral health care reimbursement over the next 5-10 years. In his words, “AI and machine learning (ML) are now poised to radically alter the ways we provide and pay for health care. The coming changes are accelerating, their consequences are unknown, the potential benefits are enormous, and the corollary risks are speculative. The only certainty is that in five years, the ways we provide and pay for health care will substantially change.”
Eric (Alliance for Addiction Payment Reform): Matt, thanks so much for meeting with me regarding this rapidly evolving technology and the subsequent impact it will likely have on the way we approach behavioral health care reimbursement. First, can you take a moment to describe the conceptual framework that you’ve developed for examining the potential impacts of AI on behavioral health (BH) treatment and reimbursement?
Matt (McCreight Partners): That AI is going to impact reimbursement and the BH treatment industry is not a question – only “when, where, and how” is. Since AI’s potential impacts run the gamut from purely mechanical to existential, I’ve found it helpful to break the issues down into three categories: Operational, Behavioral, and Philosophical (OBP). The use of these three categories can help organize our thinking around the “when, where, and how” dilemmas we face in assessing AI’s potential impacts on BH reimbursement.
Under the OBP framework, “Operational” refers to the nitty-gritty mechanics of delivering, tracking, and paying for care. “Behavioral” encompasses the broad range of therapeutic and motivational tools, practices, and outcomes that make up the treatment continuum and standards of care. “Philosophical” explores the more ethereal dimensions of how AI will impact human experience and its “meaning.” All three of these categories will ultimately have an impact on how we define and pay for outcomes.
Eric: How can we anticipate AI will impact us in the near and long term?
Matt: AI’s initial impacts (1-3 years) will first manifest operationally through the adoption of AI-assisted management systems to facilitate documentation, coding, tracking, reporting, billing, etc. The changes will be direct, easy to implement, simple to measure, and produce quantifiable results. The economic benefits from process reengineering and efficiency gains can be recognized quickly.
Intermediate impacts (3-5 years) will result from incorporating AI capabilities into changing treatment models and Security Operations Centers (SOCs), enhanced analytical and decision support systems, and restructuring performance management and reimbursement systems. These next-generation AI-powered changes will integrate data from a broad range of sources (sensors, predictive algorithms, pharmacogenomics, etc.) to permit continuous tracking and agile modification to both individual treatment plans and care delivery models. These dynamic programs will drive changes in SOCs that will be propelled by and reflected in reimbursement systems. The behavioral impacts of these changes will show up in patients’, providers’, and payers’ altered expectations and behaviors. The economic costs and benefits will be reflected both structurally and transactionally. Over time, they will significantly change the clinical and financial models used by the treatment industry.
The longer-term effects (5 plus years) will ultimately reveal themselves in the way individuals and societies perceive themselves in terms of what’s deemed “healthy” and “normal” versus “unhealthy” or “abnormal.” The full integration of AI will accelerate the evolution of increasingly hybrid human and AI systems where the boundaries between human and produced inputs and outputs will get blurry. AI-powered “assistants,” “companions,” and neural implants are a few of the sci-fi-ish components that lie just beyond the speculative horizon.
The actual “meaning” of the emergence of these hybrid systems in terms of human experience is unknown. How they will alter our future concepts of “identity,” “mental health,” and treatment are presently unknowable. While discussions of philosophy in science and business are generally to be avoided, the reality is that such changes to the fundamental meaning of human experience will be real and best approached with eyes wide open.
Eric: Can you go into a bit more detail as to what the defining characteristics of AI are in terms of its Operational, Behavioral, and Philosophical dimensions?
Matt: The chart below depicts the three OBP tiers with their most salient traits with the corresponding change modalities labeled: “Programmable,” “Inducible,” and “Evolvable.”
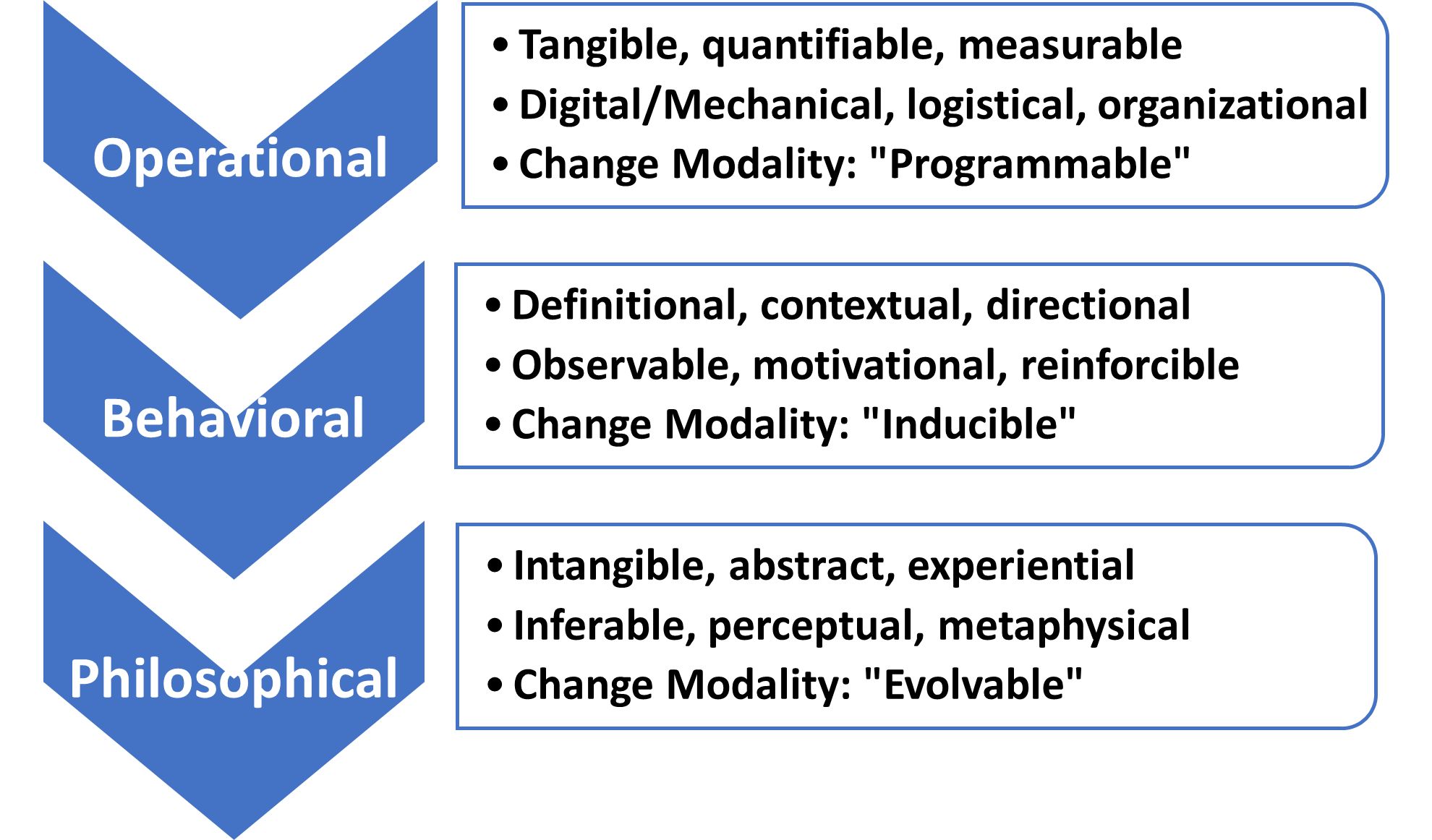
Each OBP layer is based on a different change modality that operates over different timeframes with different “drivers.” Applied appropriately, each change method can produce good outcomes with minimal friction, conflict, or unintended consequences. If ignored, misapplied, or forced, the outcomes tend to be messy, costly, and unpredictable.
The inherent nature of the three modalities is most evident in the timeframes they operate on and causality. “Programmable” methods are linked to concrete actions, implemented quickly and directly measurable.
“Inducible” methods stretch out the relationship between cause and effect. Change becomes less short-term and linear, and more like a mosaic where the outcomes have to be inferred.
“Evolvable” changes (as the name implies) emerge from cycles of programmable and inducible changes supplemented with randomness and unintended consequences. It’s as if the elements of causality have been thrown into a blender. It’s only possible to see the outcomes of evolutionary change looking backward.
Eric: Given your OBP model for AI and ML, where would you suggest that BH providers focus their initial attention over the next five years?
Matt: The obvious first choice for BH providers is to go for the low-hanging fruit. The administrative overhead burden associated with claims processing and the “revenue cycle” for BH treatment varies from 10 percent to as high as 30 percent of billed revenue. Given the antiquated nature of these systems and their Byzantine complexity, they are ripe for AI process improvement and automation. Virtually every aspect of the process flow can benefit from AI-driven reengineering and optimization. The short-term costs in investment and disruption will be more than offset by productivity gains for those who adopt AI-enabled systems appropriately.
On the flip side, it’s important to recognize that since the potential gains are so large, AI represents both a competitive opportunity and a liability to providers and payers. Providers who successfully embrace these new tools will improve their efficiency, outcomes, and profitability. Those that don’t will be disadvantaged on all three levels. Similarly, payers who embrace these new tools will be able to reduce the cost of claims processing, increase fraud prevention, and improve risk management.
In the eternal, and at times adversarial, tug of war between providers and payers, an imbalance of AI capabilities on either side will have potentially challenging consequences for the other.
Thinking positively, AI process improvements have the potential to benefit all three segments of the BH treatment industry: providers, payers, and patients. Applied appropriately, AI can eliminate administrative burdens that convey no therapeutic benefits and reduce access to care.
Eric: From a member/patient perspective, how will advances in AI and ML impact their experience with the BH service delivery system?
Matt: Ultimately, the members/patients stand to be the greatest beneficiaries of AI adoption, assuming it is done appropriately. Besides reducing administrative costs and removing access barriers, AI offers the prospect of dramatically improving our ability to provide personalized medicine. From initial diagnosis through all the phases of treatment planning and care management, AI tools can assist providers in the personalization of treatment. AI can match individual genetic profiles with Medication-Assisted Treatment (MAT) administration, personalize the timing and use of different types of therapy, identify positive and adverse responses to different therapeutic regimens, and monitor long-term relapse prevention. AI can be used to match individual patients with specific treatment programs and providers. And AI can supplement direct human intervention with AI tools and “agents” that can enable patients to become more active participants in the development of their personal recoveries.
That said, AI has its downsides. Privacy concerns (HIPAA), training set “bias,” the danger of decision support becoming “decision default,” and the potential, if misapplied, to further de-humanize and alienate people who are desperately seeking healthy human connection are all real liabilities if AI is not incorporated thoughtfully.
Eric: Are there any final considerations you would like to pass along to our Alliance for Addiction Payment Reform participants?
Matt: AI’s impacts on BH treatment practices and reimbursement are not “coming.” They’re already here. They’re being felt at all three OBP levels. It’s important to recognize that at the time of this interview, it’s only been a year since ChatGPT burst on the scene, and all the elements we’ve been discussing have emerged from obscurity to become hot-button issues. In the context of the OBP framework, they’re already coming in ways that make it difficult to recognize, isolate, understand, or predict specific outcomes with certainty.
There is no question that AI offers the BH treatment industry enormous potential benefits that will alter the way we define treatment models, measure outcomes, and provide reimbursement. There’s much about AI related to treatment and reimbursement, so we should be hopeful as long as we step carefully.
So, in conclusion, I’ll offer two pearls of wisdom from that great sage Yogi Berra opining on the pitfalls of undertaking journeys of this urgency and magnitude: “We’re lost, but we’re making good time” and “If you don’t know where you’re going, you might wind up someplace else.” Let’s get it right!
Eric: Matt, thanks for your thoughtful responses to this important conversation. Your comments have given us a great deal to consider when considering AI and ML’s impact on behavioral healthcare reimbursement.
The Alliance for Addiction Payment Reform (Alliance) is a national multi-sector alliance of health care industry leaders – including payers, health systems, recovery service providers, and subject matter experts – dedicated to aligning incentives and establishing a structure that promotes the type of integration and patient care capable of producing improved outcomes for patients, payers, and health systems. The Alliance brings together clinical, addiction, information technology, primary care, social, regulatory, and policy expertise and has logged hundreds of hours of workgroup meetings, ratifying consensus principles and outputs.
McCreight Partners (www.mccreightpartners.com): McCreight Partners is committed to partnering with our clients to ensure their sustainable competitive superiority. Our practice profile offers a consolidated look at our firm’s client-focused, outcomes-driven practices. Our engagements speak to the competencies of our global Alliance Partner Network and Research & Operations Center (ROC) professionals, from education and healthcare to energy and national security. We focus on seven sectors of the economy. McCreight Partners reflects the clients we serve, our professionals, and our work.